Effective Intrusion Detection System Using Deep Learning for DDoS Attacks
DOI:
https://doi.org/10.62019/abbdm.v4i1.113Abstract
An increasing demand for information technology and cloud computing has led to various security threats over internet. Amongst all types of threats, Distributed Denial of Service (DDoS) attacks are the most common, posing a challenge to a cyber security. It allows unauthorized users to gain access to services, and preventing authorized users. Traditional intrusion detection, antivirus software, and firewalls are not enough to detect these attacks. This paper proposed an efficient deep learning-based intrusion detection system (IDS) for DDoS attacks detection. Proposed model is a hybrid CNN-LSTM model which is combination of best DL algorithms to take advantage of CNN to extract spatial features and LSTM to extract temporal features. The real-time DDoS-specific benchmark-dataset CICDDoS2019 used for training, testing, and validation. An autoencoder has been used for dimensionality reduction, and SMOTE method is used to balance the minority class of dataset. The experimental results proved that the model outperforms than other state-of-the-art models, with an accuracy score of 99.86%.
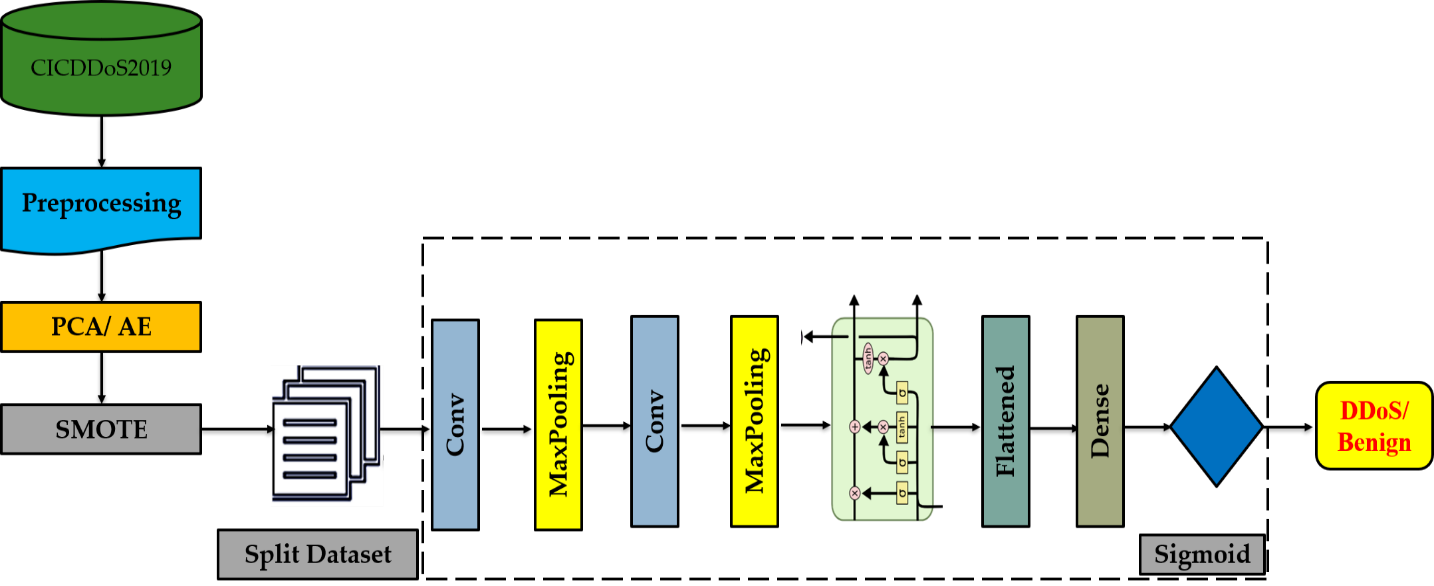
Downloads
Published
Issue
Section
License
Copyright (c) 2024 The Asian Bulletin of Big Data Management

This work is licensed under a Creative Commons Attribution-NonCommercial-NoDerivatives 4.0 International License.